© Pint of Science, 2025. All rights reserved.
Deep learning invades ecology – Computer vision in monitoring flowering on Alpine meadows
Mikko Tiusanen
(Post-doctoral researcher, ETH Zürich)
The increasing digital data and evolution of algorithms enables us to analyze our surroundings, e.g., society, space, and nature, with improved precision and scales. This provides opportunities to improve our understanding of phenomena within many fields of science, including biology.
Climate warming is shifting the seasonal timing of species’ life history events. Still, our understanding of these effects remains poor, especially within complex natural communities. Here, for a higher definition picture of alpine flowering communities, we monitor them with camera traps. To evaluate how flowering reacts to changes in temperature, we use a machine learning algorithm in species identification.
Climate warming is shifting the seasonal timing of species’ life history events. Still, our understanding of these effects remains poor, especially within complex natural communities. Here, for a higher definition picture of alpine flowering communities, we monitor them with camera traps. To evaluate how flowering reacts to changes in temperature, we use a machine learning algorithm in species identification.
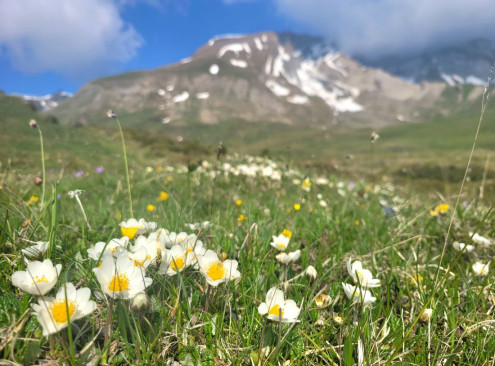
© Mikko Tiusanen
Educating smarter AI to facilitate ecological research
Hongyuan Zhang
(PhD student, ETH Zurich)
Cell segmentation models must be generalizable to handle diverse biological contexts, while model predictions require verification and correction for accurate statistical reasoning. Therefore, when a domain-generalized model is available and tools can readily refine its initial predictions, the revised results from the research community could serve as a diverse training source. This iterative process improves model performance and reduces manual corrections. We refer to this as the community-driven human-in-the-loop approach, and we have developed a series of resources to realize it. Specifically, in the stomatal segmentation domain, we provide the most diverse and extensive training dataset along with the corresponding vision foundation model through parameter-efficient finetuning. Additionally, we have developed an annotation tool that integrates the Segment Anything Model series to facilitate prediction corrections. Our testing results across multiple labs worldwide demonstrate their effectiveness, which can be applied to other domains.
Map data © OpenStreetMap contributors.
Other Sein events
2025-05-19
Eyes Wide Open
Sein
Hohlstrasse 355 8004, Zürich, Switzerland
2025-05-21
Equations in Action
Sein
Hohlstrasse 355 8004, Zürich, Switzerland